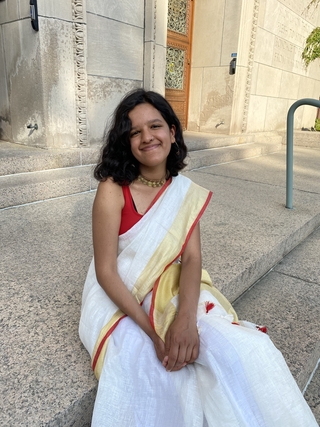
Speech production and perception have often been studied in a non-intersecting way, and experiments are restricted by methodological and analytical constraints (Casserly & Pisoni 2010). For example, the study of these two processes often makes use of different dependent measures, such as articulator movement properties for production versus discrimination accuracies or reaction times for perception. However, there is also consensus that these two processes must have overlapping cognitive mechanisms that underlie them. The theories that linguists pursue reflect this consensus: for example, be it the phoneme, motor movements, or gestures, it is agreed that production and perception must have some shared representation in play. This dissertation will propose a combined neural model of perception and production couched in dynamic field theory (DFT). The model will be constrained by three empirical phenomena: compensation in response to perturbed auditory feedback, convergence to an interlocutor, and perceptual learning. The consideration of these three phenomena together has the potential to uncover new insights about the cognitive mechanisms that overlap in speech production and perception. As a starting point towards a unified model, we will consider two potential areas of overlap. We will pursue the hypotheses that 1) The efference copy, proposed to explain compensation to perturbed feedback, also plays a role in phonetic convergence. Instead of being static, we propose that it varies according to a talker’s goals in context. And, 2) Perceptual learning, proposed to explain listener adaptation in context, also applies to production targets, informing this context-specific efference copy. Perceptual learning is thus the label given to a manifestation of a more prevalent process. Taken together, these hypotheses suggest a deeply integrated cognitive system jointly underlying speech perception and production. Making the links between perception and production precise, in the context of these three phenomena, will allow the specification of a model that generalizes beyond them.